Mitchell Lovett
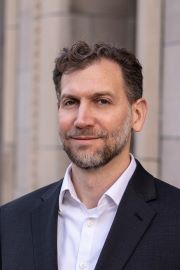
Mitchell Lovett
Professor Lovett is the Senior Associate Dean of Education and Innovation. He is also a leading scholar and teacher as the Benjamin Forman Professor of Marketing. He joined the Simon Business School in 2008 after earning his PhD in marketing from Duke University. In his administrative role, he has been instrumental in launching the AI Initiative, a cross-disciplinary effort to integrate AI into Simon’s business education. He also helped to develop the Online Masters in Business Analytics and Applied AI, a cutting-edge program that prepares students for the rapidly evolving data and AI-driven economy. His research interests span a wide range of topics in marketing, such as advertising, branding, word-of-mouth, political marketing, consumer and firm learning, retailing, and conjoint analysis. He applies and develops empirical methods to study marketing phenomena and to inform marketing decisions. His research has been published in top journals and garnered recognitions such as the Marketing Science Institute's Young Scholars and Scholars distinctions, and the William F. O'Dell award finalist for long-term impact. His research has also attracted national media attention, and he has been cited in outlets such as the New York Times, Forbes, and Ad Age. He is frequently invited to speak at academic and industry conferences and events. He also advises PhD students and is an award-winning teacher including courses on marketing research, marketing strategy, analytics design and applications, advertising strategy, consumer behavior, and PhD seminars in quantitative marketing.
- Courses
-
Advanced Marketing StrategyCore Research Topics In Quantitative MarketingAnalytics Design & Application
- Research Interests
-
Professor Lovett's research interests include quantitative marketing, retail strategy, targeted advertising, advertising content and schedule choices, online and offline word-of-mouth, branding, social media listening, and consumer learning. One stream of Professor Lovett's research focuses on applying and developing empirical methods for political marketing. Current projects in this stream study the dynamics behind why candidates go negative in their political advertising, how candidates can improve their targeting of political ads, and the role of advertising versus social media in influencing voter sentiment. A second stream of research examines entertainment products and how consumers learn about them as they decide whether to continue engaging. Another current stream of research examines how advertising and brand characteristics influence word-of-mouth online and offline and how these two channels differ. Professor Lovett's research has been published in Marketing Science and the Journal of Marketing Research, received research grants and awards, including the from Marketing Science Institute Institute for the Study of Business Markets’ Research Grant Silver Medalist Award, and garnered national media attention in relevant publications such as Ad Age and Marketing News.
- Teaching Interests
-
Professor Lovett has taught Advanced Marketing Strategy, Marketing Research, Marketing Strategy, Advertising Strategy, Consumer Behavior, and PhD Seminars in Quantitative Marketing.
- Publications
-
Disentangling the Effects of Ad Tone on Voter Turnout and Candidate Choice in Presidential Elections2021Management ScienceEmpirical Research on Political Marketing: A Selected Review2019Consumer Needs and SolutionsIssue3Volume6Can Your Advertising Really Buy Earned Impressions? The Effect of Brand Advertising on Word of Mouth2019Quantitative Marketing and Economics / SpringerIssue3Volume17Product Launches with New Attributes: A Conjoint-Consumer Panel Technique for Estimating Demand2019Journal of Marketing ResearchIssue5Volume56Mobile Diaries Benchmark Against Metered Measurements: An Empirical Investigation2018International Journal of Research in MarketingIssue2Volume35The Role of Paid, Earned, and Owned Media in Building Entertainment Brands: Reminding, Informing, and Enhancing Enjoyment2016Marketing ScienceIssue1Volume35Targeting Political Advertising on Television2015Quarterly Journal of Political ScienceIssue3Volume10A Dataset on Brands and their Characteristics2014Marketing ScienceIssue4Volume33Optimal Admission and Scholarship Decisions: Choosing Customized Marketing Offers to Attract A Desirable Mix of Customers2012Marketing ScienceIssue4Volume31Marketing and Politics: Models, Behavior and Policy Implications2012Marketing LettersIssue2Volume23Seeds of Negativity: Knowledge and Money2011Marketing ScienceIssue3Volume30