The power of the generalist
The power of the generalist
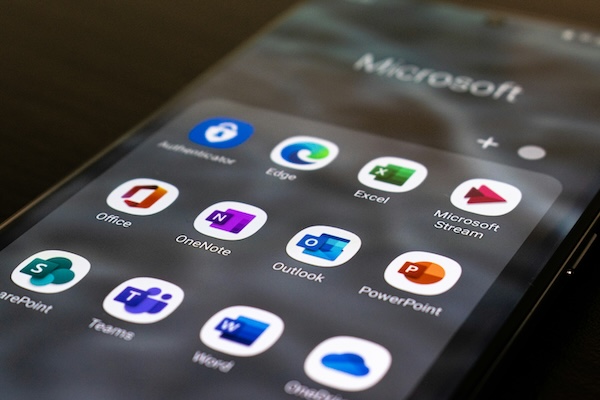
The power of the generalist
March 13, 2024 | By Dean Sevin Yeltekin
In this Q&A, technology strategist Aditya Singh’08S (MBA) reflects on how generative AI is changing the world of work and predicts who will succeed in the new normal.
Sevin Yeltekin: What brought you to Simon?
Aditya Singh: I moved to the U.S. to complete a master’s degree in computer science and found myself at a crossroads. I could become a subject matter expert and lean into more technical work, or I could move into a business role and help clients leverage technology to achieve their goals. I found the business path more appealing, which is how I ended up at Simon to pursue an MBA. I naturally gravitated toward Simon’s data-driven, analytical approach because of my background. What I found was that Simon doesn’t just give you a skillset—it teaches you to think in a certain way. Being called upon to solve problems you know nothing about creates discomfort that leads to growth. That is how you thrive in future positions.
SY: Now you’re a leader in technology strategy at Microsoft. What does your role entail?
AS: Within Microsoft’s Financial Services Industry sales team, my role is to help clients leverage our full technology suite to achieve their goals. I am a generalist, not a deep subject matter expert. My job is to put all the technology in front of the client like pieces of a Lego set and help them build something meaningful.
SY: When did generative AI first come onto your radar, and how is it changing the nature of your work?
AS:I was introduced to the concept of AI in the early 2000s when I was doing my master’s degree. I was familiar with models that could be trained to make inferences, but everything changed when companies like Open AI began training Large Language Models (LLMs) with sweeping applications. Now we have a tool that doesn’t just scour transport data or climate data; it is trained on the entire universe of written language. At Microsoft, I have the privilege of trying new technology before it is commercialized, so there are plenty of generative AI tools that are still in development. But I can say that I currently use M365 Copilot, our AI assistant, to do things like produce meeting transcripts and set reminders. Generative AI is particularly useful for noticing things that happen on a schedule, like when someone sends an expense email every month around the same time. The AI tool might take notice and ask to automate it. If you say yes, that’s one more task off your plate for the day. This is just one example of the many repetitive tasks that AI will soon take over.
SY: How should we fill the time saved by using generative AI to do more routine tasks?
AS: As time goes by, generative AI will leave humans more productive for things that matter. There will certainly be more opportunities for deep, strategic thinking. But that doesn’t necessarily have to take place in an office. We are all better when we have space to let our minds roam—whether that looks like thinking through a client’s problem on a long walk or spending more time with family. AI creates less pressure to do mundane tasks, but the answer is not to replace every mundane task with another task. That’s not good for people and their organizations in the long run.
SY: How are your clients using generative AI to enhance their work?
AS: My clients in the financial services industry are using generative AI to tackle a broad range of problems. I often help them leverage our tools to improve employee productivity and reduce communication barriers on platforms like Outlook, Teams, and PowerPoint, where people spend most of their workday. My clients are also looking to embed the power of LLMs into very complex systems so they can in turn serve their own clients more effectively. A lot of this is not visible to the public—it is part of their secret sauce. Overall, I have noted an eagerness in my clients to understand how competitors are using generative AI. This desire to maintain a competitive edge is driving adoption and innovation in an industry that can be slow to evolve. Nothing happens with the snap of a finger, but as AI tools are gradually baked into day-to-day work, people notice colleagues trying them and decide to take the plunge themselves. Then, over time, the entire culture changes.
SY: When it comes to generative AI, what kind of skills would you want a new hire to bring to the table?
AS: Generative AI is changing the entire fabric of the global workforce. It will create new industries and require new skills in ways we can’t even predict. But what will stay the same is the kind of employee that companies like Microsoft want to hire. It is the generalist, the person who is trained in multiple areas and has multiple skillsets, who brings the most value to the table. Generalists will combine the power of AI with the weight of their varied, rich experiences to solve problems in a way that a machine never could. When it comes to being nimble and adaptive in solving out-of-the-box challenges, there is still no match for the human mind.
Sevin Yeltekin is the Dean of Simon Business School.
Follow the Dean’s Corner blog for more expert commentary on timely topics in business, economics, policy, and management education. To view other blogs in this series, visit the Dean's Corner Main Page.