5 Questions about algorithmic pricing and competition
5 Questions About Algorithmic Pricing and Competition
May 9, 2023 | By Jeanine Miklós-Thal
As the director of the Business & Policy Initiative at Simon Business School, I am fortunate to have a front row seat to timely, engaging presentations by some of the brightest minds in business and policy.
On March 30-31, the Business & Policy Initiative hosted an Antitrust Economics & Competition Policy Workshop that featured a roundtable event on algorithmic pricing and competition. When it comes to topics that are catching the attention of antitrust regulators and scholars alike, this one is at the very forefront.
Three antitrust experts served as panelists at the roundtable: Ai Deng (Charles River Associates), Joe Harrington (Wharton Business School), and Nathan Wilson (Compass Lexecon).
Below, I summarize the roundtable discussion of five pivotal questions surrounding algorithmic pricing and competition:
What is algorithmic pricing, and what are some common concerns surrounding its use?
(Jeanine Miklós-Thal): Algorithmic pricing is a broad term that refers to automated decision-making in price management. Pricing algorithms can take many forms, from simple rule-based algorithms to sophisticated artificial intelligence algorithms, but the common feature is that prices are updated automatically at high frequency based on data about factors like competitor prices or demand and supply conditions.
Algorithmic pricing has become more common over the last decade thanks to the availability of big data and the rapid advances in AI and machine learning. This rise in algorithmic pricing, while promising efficiencies in price management, has led to some concerns about potential adverse effects on competition. One concern is that algorithmic pricing might lead to more collusion, either because algorithms help humans implement collusive agreements, or because autonomous algorithms learn to play collusive strategies. Another concern is that algorithms might cause higher average prices even in the absence of collusive strategies being played.
Considering what we currently know about the effects of pricing algorithms, is harm to competition likely?
(Ai Deng): A research paper that came out in 2020 shone a spotlight on the possibility of autonomous pricing collusion without any human intervention. While this makes sense in theory, algorithmic design matters. My reading of the more recent literature is that there isn’t convincing evidence that a single-minded, profit-maximizing algorithm would learn to collude without human intervention that is designed to achieve that goal. That said, research does show that algorithms affect pricing in several, sometimes subtle ways.
(Joe Harrington): In terms of self-learning algorithms, the threat is not imminent. There is a tremendous gap between what these algorithms and what humans can do. The Q-learning algorithm, for example, takes tens of thousands of rounds of calculations for collusion to emerge. Humans in a lab will not always collude, but they can certainly do so within 15 to 20 rounds. And it might only take one price for humans to think that a competitor is trying to tell them something and decide to match it. That does not happen with AI.
(Nathan Wilson): The literature has shown that the use of pricing algorithms does seem to change what is being sold at what price and in what quantity. So individual firms and buyers are being impacted. But from an antitrust perspective, is adoption of this technology shifting conduct parameters across industries? I am less convinced that this is the case.
Pricing algorithms are often designed and sold by third-party firms that have their own incentives to make profit. What are the implications of this for how algorithms will be designed and how their adoption will affect competition?
(Joe Harrington): Compared to self-learning algorithms, third-party algorithms do seem like more of an imminent threat. No third party is exclusively designing pricing algorithms for the purpose of collusion, but anti-competitive concerns are there.
See some examples below:
UK Competition & Markets Authority: “If a sufficiently large proportion of an industry uses a single algorithm to set prices, this could result in ... the ability and incentive to increase prices.”
OECD: “Concerns of coordination would arise if firms outsourced the creation of algorithms to the same IT companies and programmers.”
There is some tentative support for these concerns. Third parties themselves have made pronouncements that their algorithms will prevent price wars. And we know that they have different incentives than companies designing their own pricing algorithms. One critical research question to explore is whether or not there is an incentive for third parties to design algorithms in a way that makes prices less competitive.
(Nathan Wilson): I do think the rise of third-party algorithms is a potential concern. The human capacity to figure out ways to coordinate is extremely impressive. On the other hand, the expectation that we’re operating in a highly competitive paradigm that might be disrupted by the adoption of pricing technology doesn’t necessarily make sense to me. I think in a lot of circumstances we’re not in a terribly competitive market. When I worked at the Federal Trade Commission (FTC), we noticed players who might not go after Big Customer X with the expectation that their competitor won’t go after Big Customer Y. Will pricing algorithms make a difference in the level of competition in these sorts of markets? It is extremely hard to say.
Do we need new legal standards and new enforcement tools to deal with the risk of algorithmic collusion?
(Joe Harrington): Tacit collusion by humans is legal because there is no remedy. Tacit collusion by AI is currently lawful but there may be a remedy. We could restrict algorithms in a way we can’t restrict human managers. But any action is premature, because we need to know more about how these algorithms work before we can think about restricting them. Right now, people are concerned about algorithmic bias and discrimination—for example, algorithms engaging in gender and racial discrimination in the process of making decisions about who gets approved for a loan or an insurance policy. There is a notion that we want algorithms to respect our laws and concept of fairness. The same thing is true with algorithmic pricing. We could better define collusion and make algorithms adhere to a policy of not colluding. But we need to learn a lot more before venturing into law and policy.
(Nathan Wilson): We should be hesitant about changing antitrust laws to focus on algorithmic pricing in particular. That doesn’t mean we take that option off the table indefinitely. I just don’t know if we can be confident enough that harm is likely to write new laws that will be difficult to change and may have adverse effects in other ways. We should also note that typically, changes that impact supply and demand lead to a new equilibrium as new firms enter and adopt new technologies.
(Ai Deng): I concur with Joe and Nathan on this point. Speaking not as a lawyer but as a practicing economist in litigation consulting, I think it is reasonable that if a third-party developer is explicitly developing a collusive pricing algorithm, it would be held liable under the
existing antitrust law. And I encourage companies to better understand the pricing algorithms they adopt and ask how they are helping them achieve their goals, especially when their competitors also use the same algorithm.
Regarding enforcement, do you think algorithms and machine-learning can serve as useful tools for competition authorities to detect collusion or to aid authorities in other areas of enforcement?
(Ai Deng): Absolutely. I am seeing more and more competition agencies at least entertaining the possibility of using these tools.
(Nathan Wilson): A good initial screening is the most obvious way of using these tools. At the FTC, there was a gasoline price monitoring project that applied a simple quantitative screen to flag data for further investigation. For a low cost, it provided information to policymakers that might be usable. I see no reason to think that they can’t apply similar but more sophisticated tools to monitor industries with a lot of available data.
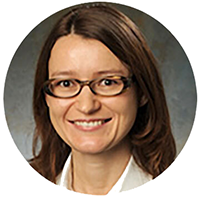
Jeanine Miklós-Thal is a professor in the Economics & Management and Marketing groups at Simon Business School. Her research spans industrial organization, marketing, and personnel economics.
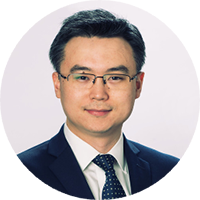
Ai Deng is a principal in the competition practice of Charles River Associates, a leading global economic consulting firm. He is also a lecturer at Johns Hopkins University and an editor of American Bar Association’s Antitrust Magazine Online.
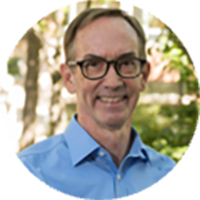
Joe Harrington is the Patrick T. Harker Professor of Business Economics and Public Policy at the University of Pennsylvania’s Wharton School of Business.
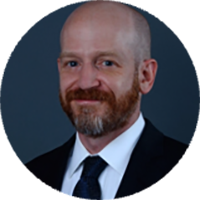
Nathan Wilson is an executive vice president with Compass Lexecon, a leading global economic consulting firming. Prior to joining Compass Lexecon, he spent 11 years at the Federal Trade Commission.
Follow the Dean’s Corner blog for more expert commentary on timely topics in business, economics, policy, and management education. To view other blogs in this series, visit the Dean's Corner Main Page.
Add new comment