AI, Competition, & Antitrust: Navigating the Complexities
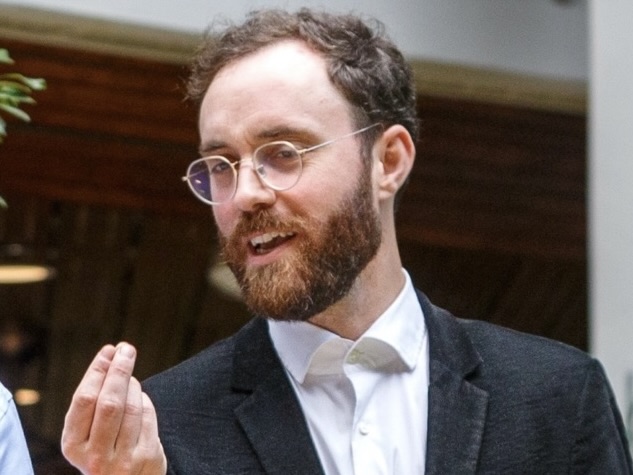
AI, Competition, & Antitrust: Navigating the Complexities of Regulation
January 15 | By Dean Sevin Yeltekin
This post recaps a recent keynote at a conference hosted by the Bradley Policy Research Center at Simon Business School.
Generative AI has immense potential to reshape industries, disrupt established players, and drive innovation. As AI capabilities grow, so does the need for regulation, particularly concerning competition and antitrust issues. The conference, "AI Policy and Regulation," hosted by the Bradley Policy Research Center, explored these challenges.
The event featured a keynote address by Thibault Schrepel, associate professor of law at the Amsterdam Law and Technology Institute. Schrepel shared insights into the complexities of regulating AI, questioned long-held assumptions about market dynamics, and offered a fresh perspective on how competition agencies—such as the Federal Trade Commission (FTC) in the U.S. or the European Commission in the EU—might approach this transformative technology.
The Evolving Landscape of AI and Competition
Professor Schrepel posed a critical question: Will generative AI disrupt the dominance of major tech companies, or will it solidify their market power? Historically, competition agencies have struggled to keep pace with the rapid rise of big tech players like Google, Amazon, and Facebook. However, with the advent of AI, regulators seem more proactive.
Schrepel noted that regulators initially approached blockchain cautiously due to its complexity and potential for unintended consequences. In contrast, they have taken a more confident stance on AI, particularly when partnerships or practices could consolidate market power.
Misconceptions of AI Competition
Schrepel addressed several misconceptions about competition in the AI space:
- Data Accessibility: Large tech companies are often assumed to dominate AI because of their access to vast data troves. However, smaller companies are finding ways to compete. Open-access databases, often available free of charge, provide valuable resources for training or improving AI models.
New techniques like generating synthetic data—a method of producing high-quality, artificially generated datasets—are also leveling the playing field. With these methods, companies no longer need massive amounts of data to succeed. - AI Models, Small vs. Large: The size of the algorithms or systems, known as "models," that are trained using this data has also raised concerns. Large AI models are more expensive to create due to higher computational power, memory, and storage needs. There is a misconception that larger AI models inherently outperform smaller ones. Schrepel explained that smaller models are increasingly capable of competing with larger models due to advancements in efficiency and computing techniques. In some cases, smaller models have even outperformed their larger counterparts, demonstrating that size is not always the key to success in AI.
- Cost Considerations: While it’s true that training AI models can be expensive, there is growing availability of venture capital for promising startups. Schrepel noted that this influx of funding allows smaller players to compete, despite larger companies’ access to more financial resources.
- Talent Acquisition: The need for top-tier talent is often highlighted as a major barrier to start-ups and smaller players, but Schrepel pointed out that smaller companies—such as Mistral AI, which has fewer than 50 employees—are succeeding in the AI ecosystem. Moreover, employees leaving major tech firms to start their own ventures are reshaping the competitive landscape.
The Interdependence of AI Ecosystem Layers
Schrepel then delved into the structure of the AI ecosystem, dividing it into three key layers:
- AI Infrastructure: This is the core layer that encompasses hardware and computational power for AI development. Companies like Nvidia and AWS supply GPUs, servers, and cloud platforms essential for training and running models, powering innovation across the ecosystem.
- Foundation Models: Examples of this layer include GPT-4 and Anthropic's offerings. They are large, pre-trained AI systems that enable diverse tasks. They bridge raw computational power and user-facing applications, allowing developers to create advanced tools efficiently.
- AI Applications: This layer is closest to end-users. Examples like ChatGPT and Claude leverage foundational models to perform tasks like conversational AI, content creation, and data analysis.
The competitive dynamics within each layer are intertwined, and improvements in one layer can have far-reaching effects on the others. For example, Google uses a neural network called AlphaChip, which falls under the Foundational Model layer, to design better semiconductor chips. These chips are the physical hardware components that power computing devices, therefore fall under the AI Infrastructure layer. This demonstrates the interconnection between hardware and software in the space.
In this scenario, software (Foundational Model layer) is used to improve the design of hardware (AI Infrastructure layer), which, in turn, supports all layers in the AI ecosystem.
Vertical integration is common in this space. Companies such as Amazon and Meta are developing their own in-house chips and infrastructure, reducing their reliance on Nvidia. As a result, competitive pressure within the GenAI ecosystem exists both within and across layers.
Increasing Returns and Market Concentration
Schrepel said two themes are critical at this stage of AI development as they relate to competition and antitrust issues. He referred to these concepts as “increasing returns” and “market concentration.”
- Increasing Returns: Factors like access to unique data, platform ecosystems, and reputation create a feedback loop. As a platform or company grows and attracts more users or developers, its value increases, which then attracts even more users or developers, creating a cycle that leads to exponential growth. This growth makes it more difficult for competitors to catch up, as the more successful a platform becomes, the greater its advantages and returns on investment.
- Market Concentration: Increasing returns have the potential to drive the concentration of market power in the hands of a few dominant companies or platforms. For example, as platforms like OpenAI’s app store becomes more valuable by attracting more developers, even more developers are drawn to it, which leads to a concentration of power and influence in that platform. This feedback loop makes it harder for smaller competitors to break in, resulting in a few companies dominating the market.
Schrepel cautioned that “historical events are not averaged away,” meaning past events, decisions, or actions have a lasting impact and are not diluted or neutralized over time. The impact of individual decisions is amplified by increasing returns. As a result, the ecosystem naturally gravitates toward concentrating market power in the hands of a few players. Schrepel concluded that this concentration can enhance consumer welfare, provided it is driven by increasing returns that improve product quality. When concentration does not improve product quality, antitrust agencies will be well-positioned to intervene.
Open vs. Closed AI Models
While some models are technically open—meaning their code is accessible—practical restrictions, such as licensing terms or non-compete clauses, can limit the extent to which the code can be modified or used commercially. Schrepel argued that true openness is crucial for fostering competition, as it allows smaller players to "fork" models and create their own versions, thereby reducing the potential for dominant players to leverage their market power unfairly.
Schrepel believes competition agencies have not yet adequately examined the terms and conditions governing AI models. He suggested these terms should be scrutinized more closely, as shifts from open to closed models could have significant antitrust implications.
Regulatory Challenges and the Future of AI
In the final portion of the talk, Schrepel discussed the regulatory challenges posed by AI. He acknowledged that existing regulatory frameworks, such as the EU’s AI Act, may inadvertently favor large corporations over smaller startups. He called for more flexible, adaptive regulations that could support innovation without stifling competition.
Schrepel argues for a nuanced, dynamic approach to regulation of the AI ecosystem. One that balances competition, innovation, and consumer benefits. The goal should be to avoid stifling innovation in the name of competition while ensuring the AI landscape remains open, diverse, and fair.
Conclusion
Professor Schrepel’s keynote offered invaluable insights into the complex intersection of AI, competition, and antitrust. His thought-provoking analysis challenges assumptions about market dominance, data, and openness, underscoring the need for careful regulatory consideration as the industry evolves.
Watch the entire keynote presentation here:
Follow the Dean’s Corner blog for more expert commentary on timely topics in business, economics, policy, and management education. To view other blogs in this series, visit the Dean's Corner Main Page.