AI, Algorithmic Pricing, and Collusion
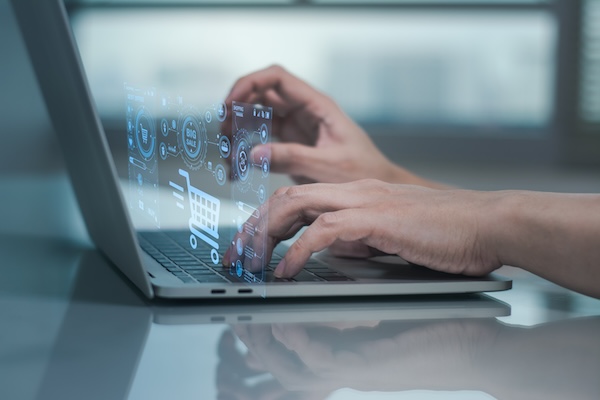
AI, Algorithmic Pricing, and Collusion
April 24, 2024 | By Jeanine Miklós-Thal
In this blog post, Professor Jeanine Miklós-Thal investigates claims that the increased use of commercial pricing algorithms could lead to more collusion.
In 2011, Peter Lawrence’s The Making of a Fly, a book on evolutionary biology, was listed for sale on Amazon for $23,698,655.93. In an early case of algorithmic pricing gone wrong, two booksellers were using Amazon’s algorithmic pricing tool to guarantee that they would earn slightly more revenue than their main competitor.
More than a decade later, algorithmic pricing is in the hotseat as scholars and policymakers express concern that it could create new opportunities for collusion. While the widespread adoption of AI and algorithms promises greater efficiencies in price management, there is rising concern that algorithms could also make it easier for sellers to charge prices that are higher than we would expect in a competitive market, either through an explicit illegal agreement or tacit collusion.
In recent research, my co-author Catherine Tucker (MIT Sloan) and I put these ideas to the test.
Types of Pricing Algorithms
Algorithmic pricing is a broad term that is used to refer to various forms of automated decision-making in price management. When analyzing the claim that algorithmic pricing could lead to collusion, the first thing to note is that not all algorithms are created equal.
It’s difficult to determine which firms are using which types of algorithms because this information is highly protected, but we know that there are two common types: predictive and rule based.
Predictive algorithms
Frequently used by larger companies, prediction-based pricing algorithms enable sellers to better tailor their pricing decisions to market conditions. These algorithms leverage AI to optimize prices based on forecasts of demand, rather than simply retrospectively analyzing historical data and calculating price sensitivities.
Rule-based algorithms
Other pricing algorithms don’t use AI at all. Instead, they automate a pricing rule, such as matching the price of a competitor, undercutting that price by a fixed amount, or charging more than that price by a fixed amount. For a modest fee, even small third-party sellers on platforms like Amazon and eBay can adopt such simple rule-based pricing algorithms.
What are the Effects of Each Type of Algorithm?
Using a variety of research methods, we analyzed the effects of these two types of algorithms—predictive and rule-based—on prices and firms’ ability to collude.
We started with the first type: predictive algorithms.
Our game-theoretic analysis models fluctuating demand due to market conditions and assumes that sellers obtain data that is useful to predict demand. Importantly, the sellers’ pricing strategies can depend on demand forecasts, in addition to the history of past prices. The primary question we were exploring was how improvements in the quality of the sellers’ demand forecast, as they switch from traditional pricing methods to pricing algorithms, affect the sustainability of collusion. In other words, we wanted to know if more accurate demand predictions made it easier or harder for sellers to coordinate on strategies that lead to high prices.
We found that predictive algorithms can actually make collusion harder to sustain.
Improvements in the quality of demand predictions accomplish two main things: First, better demand predictions allow sellers to better tailor prices to demand conditions. The sellers can implement dynamic pricing strategies more effectively when their demand predictions are more accurate.
Second, however, more accurate demand predictions raise each seller’s temptation to undercut price and steal demand from competitors at times when demand is predicted to be high. This effect can make coordination less effective.
Next, we took a closer look at rule-based algorithms. We partnered with Egor Kudriavtcev, a PhD student at the University of Rochester, to run a small-scale experiment with MBA students specialized in pricing at Simon Business School. Our goal was to understand whether price levels differ between markets in which sellers have access to simple rule-based pricing algorithms and markets in which sellers are confined to setting prices in the traditional manual way.
The participants played a real-time market simulation over two weeks on their own devices. In the simulation, each market featured three human sellers who were active on two markets: a so-called manual pricing market, where sellers had to set all prices manually, and a market in which sellers had the option of using simple pricing rules in addition to manual pricing. These rules were modeled after pricing tools offered to sellers by online marketplaces, as well as third-party repricing software tools.
We found that sellers who were given rule-based pricing algorithms successfully used these algorithms to achieve prices and profits closer to collusive levels.
The combinations of pricing rules they used led the entire market to higher prices, and sellers also made clever use of the minimum price restrictions in the rules to prevent prices from racing to the bottom.
The Bottom Line
The results of our experiment, although small in scale, suggest that certain types of pricing algorithms are more likely to raise competition concerns than others. Assessing the nature of algorithms is fundamental for understanding their implications for competition policy.
Specifically, our research indicates that rule-based pricing algorithms, which allow firms to peg their prices to those of competitors through a simple price matching algorithm, are more likely to raise concerns than predictive algorithms that improve sellers’ pricing performance thanks to better demand forecasts.
When policymakers and people interested in antitrust and competition policy express concern about algorithmic collusion, they should pause and ask themselves "What algorithm?"
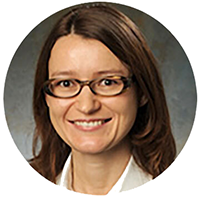
Jeanine Miklós-Thal is the Fred H. Gowen Professor of Economics and Management at the Simon Business School.
Follow the Dean’s Corner blog for more expert commentary on timely topics in business, economics, policy, and management education. To view other blogs in this series, visit the Dean's Corner Main Page.