Optimizing healthcare operations
Optimizing Healthcare Operations
June 22, 2023 | By Vera Tilson
I became interested in healthcare operations during my PhD studies in the mid-2000s. At the time, my PhD advisor cautioned me against choosing to work in such a niche field. But only a few years later, healthcare operations could no longer be considered a niche field in my academic discipline. In 2016, healthcare overtook manufacturing and became the largest industry in the U.S., employing over 20 million people. As the population ages, healthcare is projected to continue to grow.
The pressures to contain costs and the recent availability of operational data make healthcare an attractive area of research for business faculty. For the past 12 years, I have taught operations management and supervised capstone projects for Simon Business School’s Masters of Medical Management (MMM) program. Collaborating with the students working in the industry alerts me to process issues that healthcare professionals seek to resolve. I use my expertise in mathematical modeling to identify the critical factors that drive process performance and evaluate potential solutions efficiently. By analyzing the possible solutions prospectively, I can help healthcare professionals avoid waste and achieve more effective, efficient, and high-quality care.
I publish my work in journals dedicated to academic research, journals aimed at healthcare professionals, and as teaching cases. Through these publications and through presentations at academic conferences, I share the knowledge and methodologies developed at Simon with the broader healthcare industry in the U.S. and beyond.
Below, I summarize four projects I worked on recently:
1) How to efficiently use standing orders in emergency departments (EDs)?
Standing orders are symptom-specific protocols that allow non-licensed medical professionals to order medical tests or treatments when a licensed provider is not immediately available. Clinical studies on the use of standing orders in emergency departments (EDs) have produced conflicting results, with some showing that certain standing orders can decrease length-of-stay for eligible patients and others finding the opposite.
My co-authors and I study standing orders using an analytical operational perspective. We model EDs as multi-class queueing networks and identify the key parameters that determine whether standing orders can reduce ED congestion in specific settings. We show that reducing length-of-stay for some patients does not necessarily translate into shorter wait times for all ED patients.
Further, we propose a congestion-sensitive approach to initiate standing orders that can decrease ED congestion and improve the patient experience. An easily implementable congestion threshold policy allows stakeholders to decide when to use standing orders as conditions change in the ED. By using extensive simulations calibrated with real hospital data, we demonstrate that our policy can significantly reduce overall ED length of stay.
2) How can we leverage advances in technology to improve access and reduce in-office waits for appointments?
The rate of medical appointment no-shows increased after the COVID-19 lockdown period. Typically, medical providers deal with this risk by double-booking patients, which often leads to frustration and dissatisfaction for both patients and providers.
Operations researchers have suggested a myriad of ways to optimize medical appointment scheduling, but existing research assumes that appointment times can’t be updated at the last minute. When scheduling involved penciling patients in on paper and calling them on their landline, that was certainly true. But with advances in communication technology and the introduction of online (versus in-person) appointments, same-day rescheduling is more feasible.
In this paper, my co-authors and I create a dynamic rescheduling model that can adjust upcoming appointments based on no-shows. This approach can improve access by allowing physicians to schedule more patients throughout the day. It can also reduce delays and in-office waits for patients. Say you are double-booked with another patient for a 4pm slot. If there are no-shows throughout the day, the scheduling model will adjust and offer you a 2pm or a 3pm time, allowing you to avoid a longer wait if you have the flexibility.
3) When should patients be placed in an observation unit?
This paper grew out of my collaboration with Dr. Dwyer-Matzky, who was a student in our MMM program. Dr. Dwyer-Matzky was in charge of an observational unit (OU) at URMC's Highland Hospital. As flu season approached, she wanted to use historical data to create easy-to-follow guidelines for deciding which emergency department patients needed to be monitored in the OU and which ones should be admitted as inpatients. This decision is not always straightforward and has consequences for the quality and cost of care, the length of the patient's stay, and the hospital's capacity.
By analyzing data from past patients, we identified several medical conditions which frequently resulted in patients who were initially placed in the OU having to transfer to inpatient wards, increasing the length of stay and chances of errors due to handoffs. Based on our analysis, these conditions were added to the OU exclusion list.
Work on this project inspired an award-winning teaching case where we brought together three major themes: (1) Understanding the data analytics life cycle and the interface of predictive and prescriptive analytics in the context of process improvement; (2) making ethical data-driven decisions using machine learning models; and (3) effecting organizational change to operationalize the findings of the analysis.
We then expanded on this work by exploring the idea of a dynamic OU inclusion/exclusion list with real-time considerations for available capacity. This algorithm accounts for the number of beds available in the OU, beds available in in-patient wards, likely length of patient stay, and financial parameters. Our proposed algorithm can serve as an effective decision aid that objectively classifies ambiguous patient placement cases and anchors the decision-making process.
4) Do group purchasing organizations custom contracts really save hospitals money?
To save on procurement costs, most U.S. hospitals join group purchasing organizations (GPOs). In recent years, GPOs have come under scrutiny as some hospitals claimed they were able to secure lower prices by negotiating directly with manufacturers. Following a 2002 congressional investigation, many GPOs adopted a series of new practices, including custom contracts. These contracts allowed GPO member hospitals to enter into direct negotiations with vendors to improve upon the GPO-negotiated price.
To analyze whether this practice benefited member hospitals, my co-authors and I created a game theoretic model. Our model shows that allowing for custom contracts limits the benefits of demand aggregation and raises the GPO negotiated prices. As a result, hospitals that join GPOs that allow custom contracting do not come out ahead in the long run. On the other hand, hospital purchasing managers may benefit from this practice because their performance is often evaluated based on the cost savings they achieve compared to the GPO-negotiated prices.
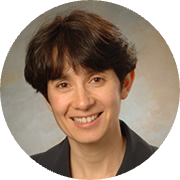
Vera Tilson is an associate professor of operations at Simon Business School. Her research focuses on healthcare operations, stochastic scheduling, and supply chain management.
Follow the Dean’s Corner blog for more expert commentary on timely topics in business, economics, policy, and management education. To view other blogs in this series, visit the Dean's Corner Main Page.